FAIRification workflow and data services
This page guides you through the typical steps for making a database FAIR. Inspired by Jacobsen et al. (2020), the workflow represents a full-fledged FAIRification cycle adapted to the needs of the low-carbon energy research community.
Access step-by-step information and FAIR data services and tools:
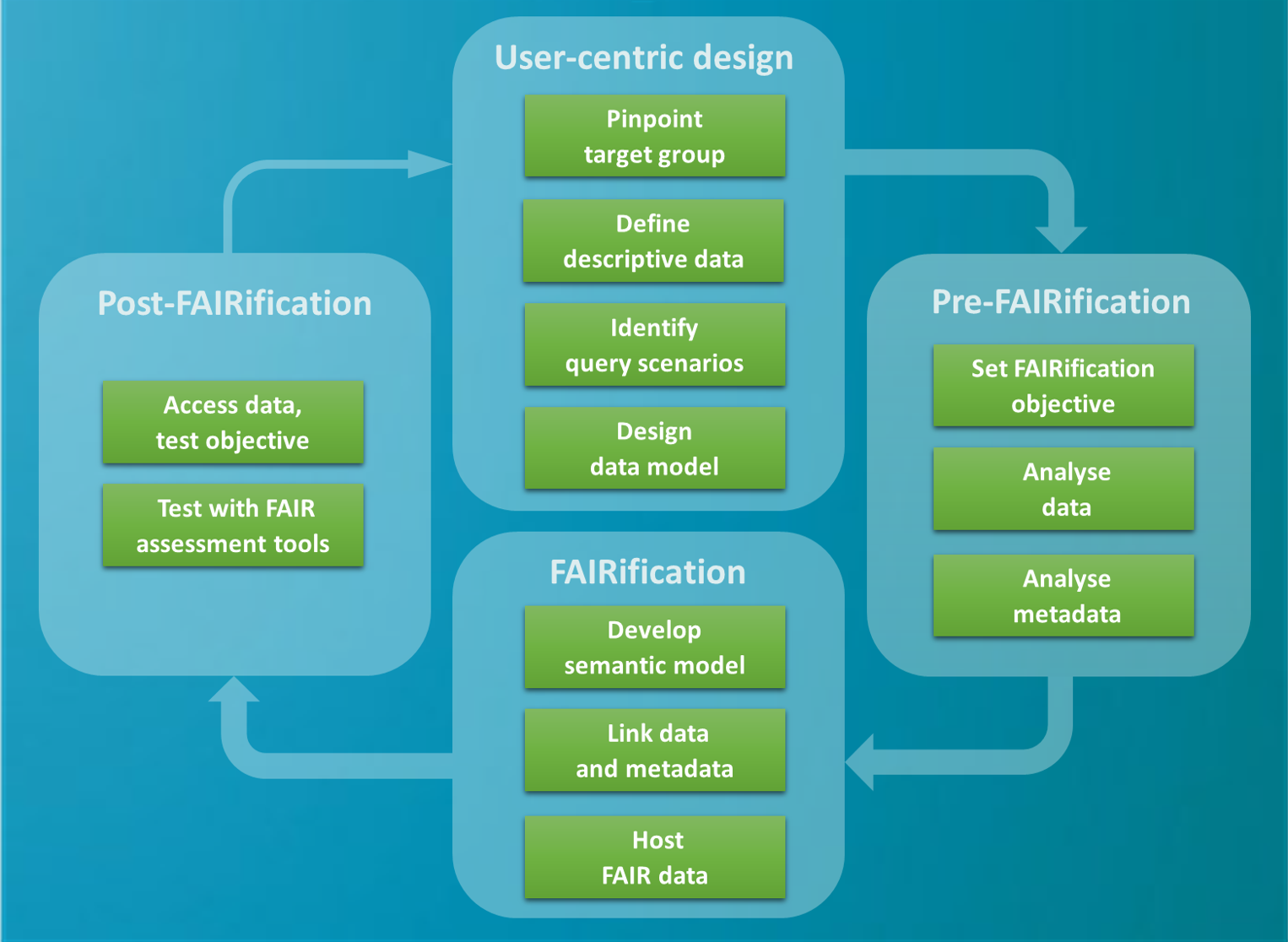
Making data FAIR requires a careful consideration of who will be the users of the data. It is important that your FAIRification strategy addresses your main target group(s) as directly as possible. They may range from academic research disciplines to the general public.
- Researchers — Energy domain experts require context information with high granularity and rich provenance to assess data quality and relevance, precise information on usage rights, and intellectual property rights (IPR) requirements. Interdisciplinary scientists need information on the context of data and provenance (mostly on aggregated levels) and precise information on usage rights and IPR.
- Science funders — Need to monitor, adjust and plan funding policies and principles to better direct R&D investments and to ensure the impact of policy measures. Typically, this application is at a high level of aggregation, and information should be easy to disseminate. A pivotal interest is that the funding agency be acknowledged in the metadata.
- Planners and decision-makers — They may reuse data, analyze some data, and publish aggregated data and decisions. This involves a middle to high level of aggregation, information on data context and provenance.
- Energy sector and other industries — Technical and operational planners and decision-makers, energy market operators draw on expert knowledge and re-use and analyze some data on all aggregation levels. They require information on context, provenance, aggregated data, as well as IPR.
- General public — The group informs itself to adjust behavior and practices. A high level of aggregation is needed to make data easy to understand and navigate.
- Data scientists — Data engineers, software and algorithm developers code, test, and validate software with existing data. They need concise metadata to integrate data sources within software tools, machine-actionable open file formats, agreed standards, terminologies, and interoperability protocols.
- Publishers, librarians, and data curators — publish, store, and archive research data. They may re-use data to link them to metrics such as access statistics and to cross-reference.
Read more on the EERAdata Wiki:
Read more on the EERAdata Wiki:
Locating data for reuse must not be reduced to the technological dimension of designing data search systems. A careful account of data seekers´ approaches has to include the socio-technical perspective to understand user practices and behaviour and ultimately to help improve the design of data discovery systems.
Read more on the EERAdata Wiki:
Data modelling is the process of creating visual representations of databases to communicate connections between data points and structures. It is the first step in making data available for the analytical needs of the users. Data models conceptually represent data with diagrams, symbols and text to visualize the associations between different data objects.
Read more on the EERAdata Wiki:
Objectives for FAIRification could be to increase the efficiency of using data from multiple sources, to meet specific requirements of publishers or funders, or to provide high quality data services for other users. It is highly recommended to do this in a team with both domain and data modelling expertise, and to provide corresponding resources. Moreover, focusing on only parts of your dataset at a time will add to the FAIRification efficiency.
The pre-FAIRification steps require i) having access to the data, ii) securing the necessary resources (regarding time and money) and iii) deciding on a realistic FAIRification plan. This step also requires a general knowledge and understanding of the data set, as well as being familiar with the FAIR data principles. These comprise a set of criteria which, in practice, are fulfilled to a greater or lesser extent, as shown by Schwanitz et al. (2022) in a recent FAIR compliance assessment.
Select one or more criteria and define your FAIRification objective for starting this workflow.
- F1: (Meta) data are assigned globally unique and persistent identifiers
- F2: Data are described with rich metadata
- F3: Metadata clearly and explicitly include the identifier of the data they describe
- F4: (Meta)data are registered or indexed in a searchable resource
- A1: (Meta)data are retrievable by their identifier using a standardised communication protocol
- A1.1: The protocol is open, free and universally implementable
- A1.2: The protocol allows for an authentication and authorisation procedure where necessary
- A2: Metadata should be accessible even when the data is no longer available
- I1: (Meta)data use a formal, accessible, shared, and broadly applicable language for knowledge representation
- I2: (Meta)data use vocabularies that follow the FAIR data principles
- I3: (Meta)data include qualified references to other (meta)data
- R1: (Meta)data are richly described with a plurality of accurate and relevant attributes
- R1.1: (Meta)data are released with a clear and accessible data usage license
- R1.2: (Meta)data are associated with detailed provenance
- R1.3: (Meta)data meet domain-relevant community standards
Read more on the EERAdata Wiki:
A proper understanding of the data is essential for carrying out any FAIRification activity. If the data are own data or coming from an in-house activity, such an understanding may come easily. But if the data are provided by a third party, a detailed analysis might be necessary.
Read more on the EERAdata Wiki:
While there is the ubiquitous statement that metadata is data about data, a more helpful definition is that metadata is information added to the data to increase its functionality. Adding information may occur during the creation but also during the whole lifecycle of the dataset. In the research context, metadata is often used to capture the context of data. This means, that all necessary information for understanding the data is described by metadata. For sharing data, metadata serves the purpose for provide this extra information so that someone else is able to work with the data given his or her own background of knowledge. But even if the data is not shared with someone else, metadata documents all relevant information so that at a later point in time the data can be used as easily as during the day of its creation. So, metadata has an important function in the scientific workflow and is closely tight to ensure proper scientific practices such as traceability, reproducibility, and transparency.
Read more on the EERAdata Wiki:
The first step of the actual FAIRification process is to assign your data with a set of metadata describing the content of the data. To improve on findability and interoperability you are advised to use existing metadata standards. The EERAdata Community Platform provides a set of standard terms from the Dublin Core Metadata Initiative and W3C´s Data Catalog Vocabulary (DCAT) relevant for energy research data.
→ Access the Metadata Creator to:
- Assign standardised metadata to your data object adhering to Dublin Core terms and DCAT V2.
- Automatically create a metadata file and add it to the searchable metadata repository on the EERAdata Community Platform.
- Download the metadata file in a machine-readable format, so that you can add it to your data resource (in JSON-LD format).
Read more on the EERAdata Wiki:
- Example on standard setting for metadata: power plant information / illustrates options for referencing to existing metadata standards
- Metadata, References on metadata
Linking data and metadata is pivotal for interoperability.
We provide an example of improving the FAIRness of a dataset using the csv extension “CSV on the web” (csvw). CSV on the web is a W3C recommendation and offers a possibility to tie together metadata and data, starting from a well-known and widely used data format. This standard offers a rich framework to annotate existing csv documents with additional information and transform them into other forms of structured data exchange formats such as JSON-LD and RDF. At the same time, CSV on the web is user-friendly offering a flexible mechanism from minimal FAIR extensions to elaborated context building for the data to be shared.
Read more on the EERAdata Wiki:
Assigning a licence to the data
Before publishing data, it is necessary to clarify the way or extent under which the data can be re-used. This is done by specifying a suitable licence in your metadata file. In the absence of a licence, the author still retains proprietary copyright, and the conditions under which the data can be used are unclear.
Standard licences provide pre-defined sets of conditions, for both providers and users. The most common licenses for a given artifact can be determined by its type: data, code, documentation, or other generic digital “creative work” (reports and figures). Special copyright rules may apply to databases, which, under European law, are protected in their own right, irrespective of the status of the data they contain.
The most commonly and widely used data licences are the suite of Creative Commons (CC) copyright licences which clearly describe how data can and cannot be reused. The CC licences are irrevocable. This means that once you receive material under a CC licence, you will always have the right to use it under those licence terms, even if the licensor changes his or her mind and stops distributing under the CC licence terms. Of course, you may choose to respect the licensor’s wishes and stop using the work, but once a dataset has been issued a CC licence, it cannot be revoked afterwards.
A scientific dataset, which other researchers may build upon or which is published together with a scientific article, is usually published under the CC-BY licence.
More on licensing and sharing
Hosting
This step is to make your metadata available to the public (and the data, if open data is supported). For publishing, several options exist such as setting up a web server with a database solution. For standalone datasets or datasets accompanying a scientific publication in a journal, the use of repositories lifts the burden of administrating a web server and offers at the same time many features supporting FAIR practices. An increasingly popular way to deploy and host FAIR data is to get involved in a suitable open data initiative (e.g., EOSC), or to upload your (meta)data to an open repository like zenodo (public) or dataverse (private).
Explore repositories through:
It is hard to achieve 100 % FAIR compliance. For this, it is important to test the FAIR criteria in practice, for instance by requesting user feedback after your FAIRification efforts.
Read more on EERAdata Wiki:
This last step of the FAIRification workflow comprises a systematic reconsideration of the FAIR data principles to validate or further improve the FAIR status of the (meta)data. If found necessary, the FAIRification can be restarted.
A number of both manual and machine assessment tools are available to achieve this, some of which have been tested within the EERAdata project in application to a large number of energy-related databases (Schwanitz et al. 2022).
- Manual assessment tools: DANS Self-Assessment Tool, ARDC FAIR Self-Assessment Tool
- Machine assessment tools: FAIRsharing Evaluation Services, F-UJI Automated FAIR Data Assessment Tool
- Examples for analyzing (meta)data in the EERAdata Use Cases